In today’s world, the combination of Metcalfe’s law and Moore’s law became a data revolution reality. Massive amounts of data are being generated from billions of smart-phones and cellular users. New 5G networks designed for Device-to-Device (D2D) communication and inexpensive sensors in the IoT (Internet of things) devices means that current human-users will soon be joined by billions of connected devices and machines.
Internet of things is here & 5G is coming
With 19 billion connected-devices today, the internet of things is already here! And 5G is almost upon us, with the test-networks to be deployed as early as 2018. 5G has been designed to add more throughput, coverage, capacity, spectral and energy efficiency and lower latency, but also more specially for manifesting Machine-to-Machine communication – low-power, efficiency, support for a multitude of devices and scale.
Velocity and size of the big data
The size of today’s data is almost unmeasurable, though challenges of collecting and transferring it around-the-world are gradually disappearing. This is occurring at the enterprise level, at the most part. The question then becomes for enterprises, and for the information officers specially, can all these massive amounts of growing data be collected in real time, processed, stored and later analyzed to support future IoT and 5G technologies?.
Such a question highlights the challenges the enterprise IT should focus on in order to consume this data explosion.
Consumption of big data
This consumption of data has to happen in real time, using distributed-messaging-layer that separates the data-capture from processing, analysis and storage of the data. Apache Hadoop and Spark bring in global event-streaming; NoSQL database capabilities and real time data ingestion. Data can be easily captured and moved in a subscribe-publish model between various software and server components.
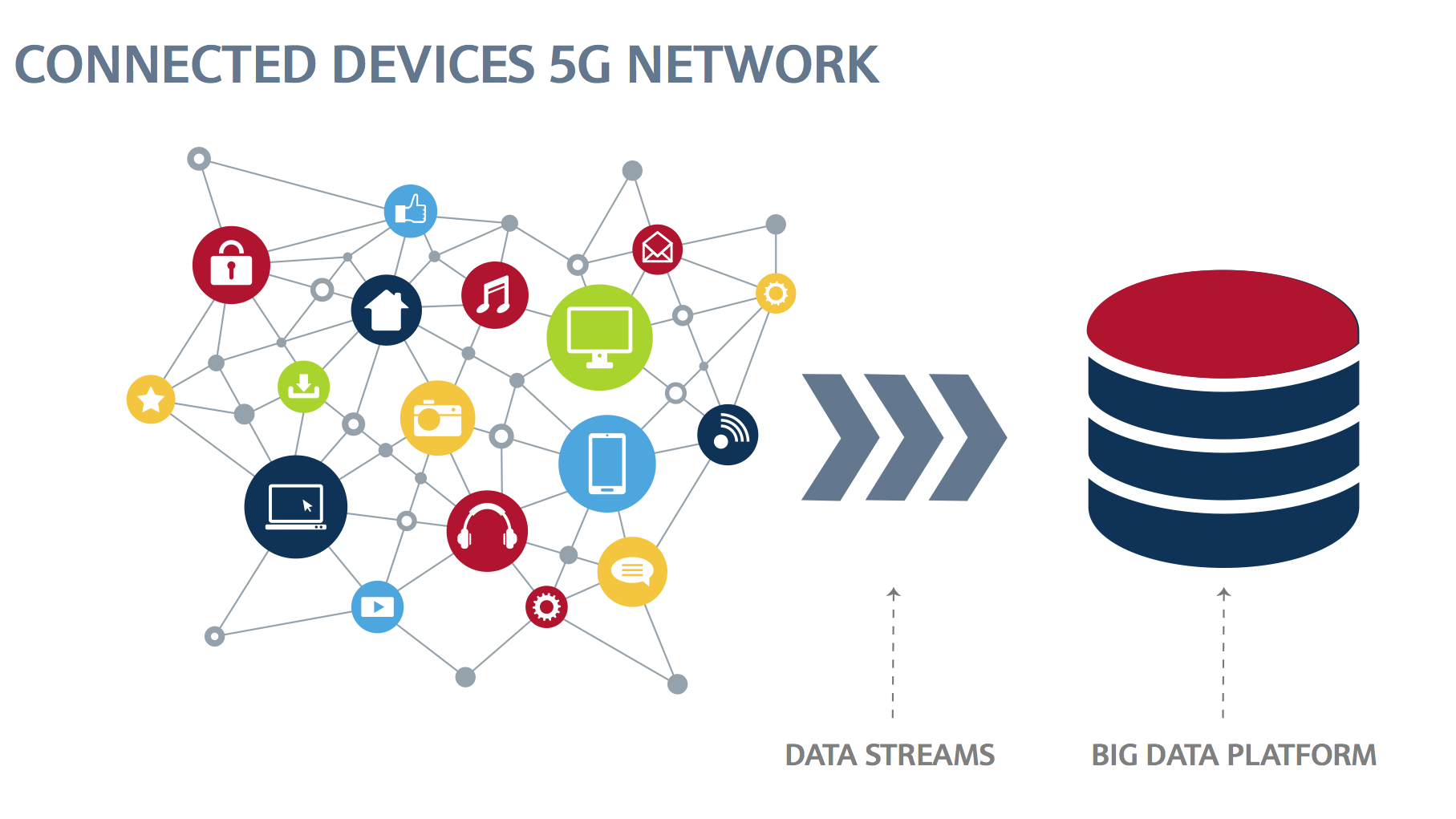
As the unstructured or raw data is streamed into storage and processing, it can be used later for filtering and aggregation for real-time analytics.
Performing rich-analytics
Apache Spark is the most used Hadoop technology to achieve this. Apache Spark can aggregate, process and transform data in a scalable and distributed way in real-time. Once you have the data processed in a scalable storage system, rich-analytics can be performed.
In the Internet of things context, which produces different formats of lots of data, the SQL engine should make a good use of the servers’ power in the cloud infrastructure and be able to deal with the different data formats in these servers. Because of its simplicity and compliance with the analytics, SQL engine, which is available in the cloud, is the most-common language for analytics. Also, the reporting tools it offers for application developers and business users makes it a reliable source. This is why cloud is essential for the success of 5G and the internet of things. It gives the enterprise the capability to analyze, store and manage these massive amounts of data flows as well as to extract information from these storage pools. Without big data and the cloud, full potential of IoT and 5G will never be realized.
To provide a scalable opendata storage platform, StoneFly formed a partnership with Microsoft that provides an unlimited file size and a scalable storage in Azure. As part of this, stoneFly has created a highly efficient and highly scalable platform in the Scale-Out NAS plugin for Hadoop. The Scale-Out NAS data storage platform brings in Hadoop and spark with global data streaming; enterprise storage for big data, real time data ingestion and NoSQL-database-capabilities.
To learn more about this subject, download the following StoneFly White paper and learn more about StoneFly’s Scale-Out NAS Cloud Storage plugin for Apache Hadoop.